In the digital era, protecting bank accounts from unauthorized access represents a paramount security imperative. Traditional username/password logins provide inadequate identity verification as data breaches continually expose credentials for criminal abuse. At the same time, modern consumers demand low-friction experiences minimizing inconvenient authentication hurdles. The experts over at Outseer.com tell us that risk-based authentication strikes an intelligent balance, enhancing safety without sacrificing convenience.
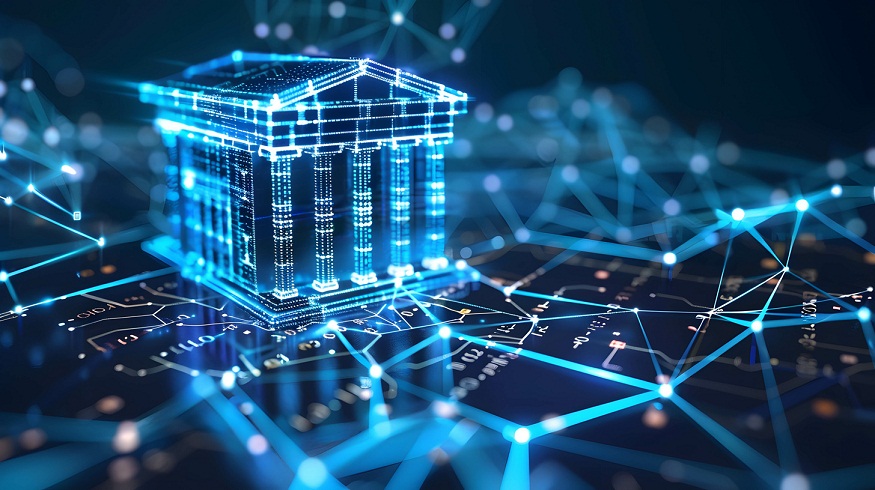
Challenges of Static Authentication
Banks have long enforced relatively static authentication policies applying one-size-fits-all login requirements like passwords plus secondary factors such as security questions or one-time codes. While strengthening identity assurance over basic passwords alone, these blanket mandates fail in accounting for risk level variances between individual sessions.
For example, logging in from a familiar device on a trusted home network presents dramatically lower fraud probabilities than an attempted login from an anonymous IP address halfway across the globe using never-before-seen hardware. Yet both scenarios faced identical authentication gauntlets, creating unnecessary friction for lower-risk events.
Conversely, overly simplistic authentication leaves ample openings when higher-risk situations arise meriting stronger identity checks. Rigid policies lack the situational flexibility required for comprehensive, yet user-friendly security in today’s world. Risk-based authentication provides that dynamic adaptability.
Contextual Awareness and Versatility
Risk-based authentication continuously analyzes a broad range of contextual variables and behavioral signals surrounding each login attempt to gauge potential risk levels accurately. It factors in variables like geographic location, network environment, device fingerprints, time of access and transaction patterns to detect signs of anomalies.
High-risk events like foreign IP addresses, unrecognized devices, suspicious transaction requests or bot-indicative behaviors trigger stricter authentication challenges like multi-factor biometrics, hard/soft tokens, or out-of-band verifications. Lower-risk scenarios like frequently used personal devices and networks allow smoother passwordless or single credential logins.
This contextual awareness flexibility intelligently tailors security experiences based on real-time situational risks rather than blanket policies. High-assurance safeguards protect higher-risk events without unnecessarily burdening lower-risk interactions, reducing consumer friction frustrations. Both security and user experience are optimized simultaneously.
Layered Identity Signal Analysis
At its core, effective risk-based authentication depends on aggregating and correlating many potential risk indicators into cohesive identity confidence scores informing steps enforced. A multi-layered approach factors data from diverse perspectives to establish more complete 360-degree visibility over users’ digital identities.
Device fingerprinting evaluates associated hardware, browser, operating system, and firmware characteristics. Geolocation tracking helps to map IP intelligence and mobile network affiliations to digital reputations. Biometrics, like facial recognition, fingerprints and behavioral patterns, introduce physical identifiers. Historical accounting activity profiles detect deviations over time.
Processing data streams through artificial intelligence and machine learning analytics engines means risk-based authentication systems synthesize holistic real-time user identity assessments from disparate signal sources. They develop more nuanced risk scoring than binary logic through advanced data correlation. Additional authentication steps only get enforced when indicated risk thresholds are breached.
Continuous Adaptation and Improvement
Risk-based authentication operates through constantly evolving trust models refining themselves iteratively through machine learning feedback loops. Models begin with baseline rules defining thresholds informed by initial data snapshots and static policies.
As artificial intelligence progressively processes more data from monitored sessions, it identifies patterns, revealing additional risk signals unaccounted for in initial baselines. Models autonomously extend their own logic to incorporate these newfound intelligence factors for augmented scoring accuracy over time.
Human teams regularly analyze outputs, optimize thresholds, and implement new model versions into production environments through governed deployment processes. This closed-circuit refinement elevates security effectiveness perpetually.
Conclusion
Overall, risk-based authentication empowers banks to provide identity proofing levels matched appropriately to real-time threats while preserving positive user experiences. Through intelligent risk assessment and adaptive challenge responses, it enhances account safety and promotes scalability as brands grow digital presences. Security and seamlessness get balance
Leave a Reply